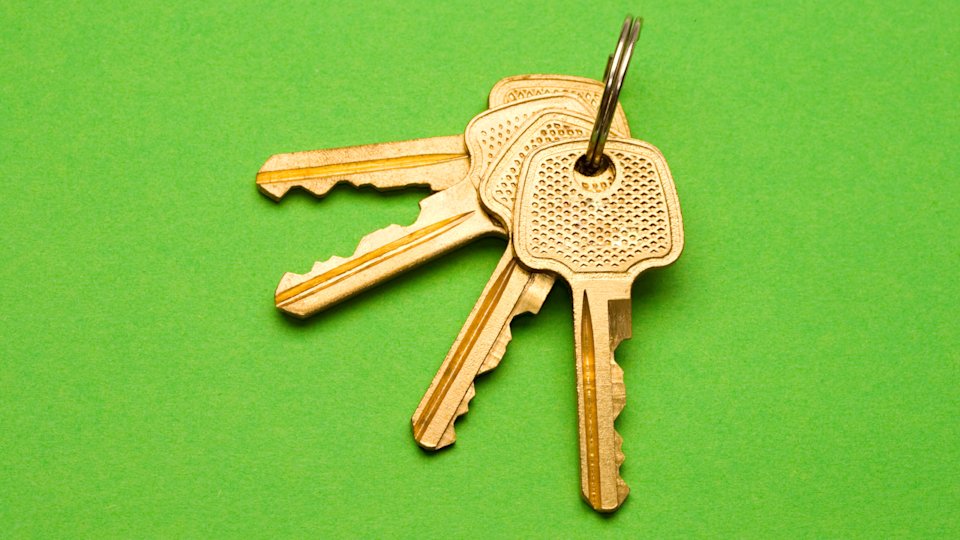
Organizations are more than the sum of their parts. A business with a great product and talented sales team won’t reach its full potential if it’s constantly drowning in paperwork and its employees don’t communicate. A basketball team full of elite players can still lose most games if the coach can’t get them to work well together.
In other words, organizations need, well…organization.
Data teams are no exception. Your data scientists, engineers, and analysts might know everything there is to know about data quality—but if their knowledge isn’t channeled through a smart, well-structured process, you’re bound to end up dealing with missing data, inaccuracies, duplicates, and plenty of other issues.
With that in mind, here are the four keys to the perfect data quality process:
1. Standardized tests and metrics
A lack of standardization leads stakeholders to work at cross-purposes. To avoid redundant work and make sure issues aren’t overlooked, your team needs to align on which metrics are most important, and which tests are best for evaluating your data against those metrics.
Say one stakeholder tests a table for accuracy and sends it to a downstream stakeholder with a message that the data has been tested and looks good. If the downstream stakeholder is concerned not only with accuracy but also completeness, which the test may not have investigated, the upstream stakeholder’s thumbs-up could be misleading.
So what kinds of tests should you look for? The five data quality tests in this article help you build shared knowledge and drive collaboration across your organization. They could be a great guide for your standardization efforts.
2. Strong documentation that’s easy to find
Once your tests are standardized across your organization, the next step is to make sure they’re well documented. There should be clear information regarding the purpose these tests serve, the information they provide, and the situations in which they come in handy.
Critically, this documentation should be easy for stakeholders to find. To that end, we recommend keeping it in a centralized and well-publicized location.
This may require some company culture management—you’ll need to enforce against bad file storage habits—but that’s easily preferable to the alternative. If stakeholders don’t know where to find information on your preferred tests, they can end up going rogue and using non-standard tests that don’t align with your organization’s goals.
3. Appropriate and accessible tools
Data quality is a concern that transcends data teams. We’ve discussed how different people at your organization may value data quality for different reasons—a data team manager, for instance, might be concerned with poor data quality affecting their day-to-day operations, while a CEO may fear the prospect of bad data leading to bad decisions that damage customer confidence.
But whatever the reason, data quality should matter to everyone.
The tools you use in your process, then, should be accessible not just to data teams but to all stakeholders in your organization. Nobody should feel that your data quality tools are too technical for them to understand or use.
An esoteric tool that only makes sense to data engineers and analysts reflects a vendor that doesn’t care about how universal a concern data quality truly is.
4. Unified, end-to-end functionality
Stakeholders at different levels of your data quality process have different needs. A data engineer can have different goals than the analysts downstream from them.
The best data quality platforms provide functionality to serve your entire process, not just one or two steps pertaining to one or two stakeholders. This offers a high-efficiency, low-risk route to a unified, end-to-end data quality process.
The alternative? Stitching together multiple resources with piecemeal functionality and hoping for the best. This not only heightens the risk of important bases not being covered, it also creates more transition points from one data system to another—each of which carries the potential for data quality issues to be introduced during transit.
Good data starts with a good data quality process. Learn how to build yours with Great Expectations.