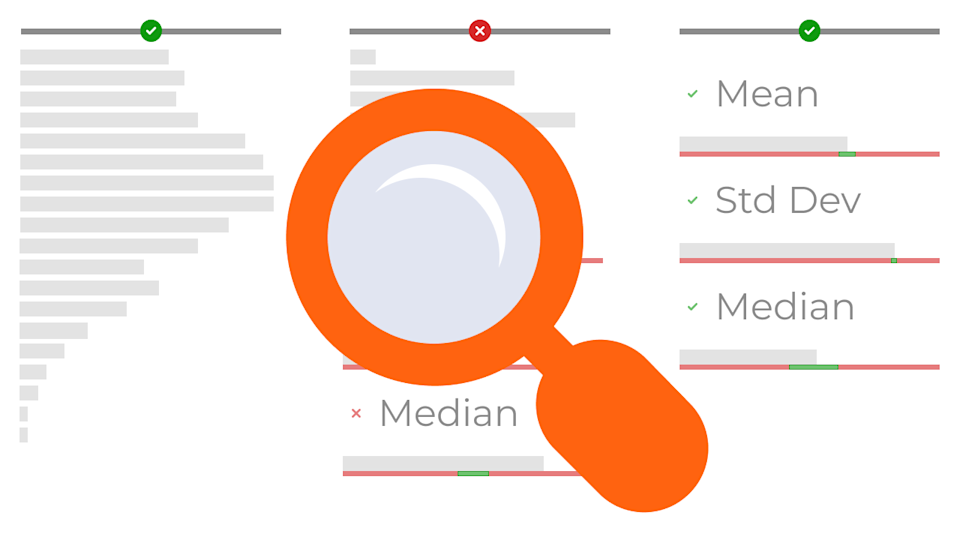
This post is the fourth in a seven-part series that uses a regulatory lens to explore how different dimensions of data quality can impact organizations—and how GX can help mitigate them. Read part 1 (schema), part 2 (missingness), and part 3 (volume).
Data quality discussions often focus on factors like volume, completeness, and consistency. But as organizations become more sophisticated in their data usage, a new dimension of data quality has emerged as a critical focus: data distribution. Forward-thinking companies are recognizing that understanding and managing data distribution patterns is key to unlocking the full potential of their data assets and maintaining a competitive edge.
📌 Quick win: Start with your most critical metrics. Before implementing comprehensive distribution monitoring, identify 2-3 key metrics that directly impact business decisions. Set up basic distribution checks for these metrics using GX Cloud's built-in Expectations. This delivers quick wins while you develop a broader strategy.
The hidden impact of distribution anomalies
As we continue the narrative of FinTrust Bank, a fictional financial institution, the data engineering lead, Samantha, is facing a challenge that might sound familiar.
Their business dashboards were showing increasingly unreliable metrics, and their financial reporting seemed off-target. The culprit? Undetected shifts in their data's distribution patterns were silently undermining their analytics accuracy.
Samantha’s team was monitoring the usual suspects—data quality, volume, and completeness. But the blind spot about how their data’s statistical properties were changing over time proved costly.
For details on how distribution analysis works in practice with GX, visit our technical documentation on distribution analysis.
Beyond basic validation: A strategic approach to distribution analysis
Distribution analysis isn't just about checking if your numbers add up—it's about ensuring your data tells the true story of your business. Leading organizations are approaching this challenge with a comprehensive strategy that encompasses several key areas.
First, they comprehensively monitor distributions, tracking key statistics across critical datasets to detect subtle shifts signaling emerging issues. They implement automated alerts for distribution anomalies and validate both column-level and row-level distributions to get a complete picture of their data's behavior.
Contextual analysis is also crucial. They consider seasonal patterns, business cycles, market events, and organizational changes affecting their data. They compare distributions across different business segments and monitor statistical validity for machine learning inputs, ensuring their analytics remain robust and relevant.
Finally, they proactively manage risk, identifying issues before they impact decisions. They maintain audit trails of distribution changes, enabling quick response to anomalies. This approach also ensures regulatory compliance through consistent monitoring, a critical concern in today's data-driven business landscape.
By adopting this strategic approach to distribution analysis, organizations can move beyond basic validation and unlock the full potential of their data, driving more accurate insights and more reliable decision-making.
Application: FinTrust Bank's journey
Samantha's team implemented a strategic distribution monitoring program using GX. They established a multi-layered approach:
1. Baseline distribution validation
Set up mean and standard deviation checks for transaction amounts
Implemented quantile validation for customer activity patterns
Established Z-score monitoring for outlier detection
2. Advanced statistical monitoring
Tracked Kullback-Leibler (KL) divergence to detect subtle distribution shifts
Monitored distribution consistency across time periods
Implemented automated checks for multimodal distributions
📌 Quick win: Leverage built-in distribution Expectations. Start with GX Cloud's prebuilt distribution Expectations like “Expect column mean to be between” and “Expect column value Z scores to be less than” to quickly establish baseline monitoring for your critical metrics.
FinTrust Bank saw a positive impact across various departments after implementing strategic distribution monitoring:
They experienced a notable reduction in false positive fraud alerts, allowing them to streamline operations and improve customer satisfaction.
Their risk model’s accuracy improved substantially, leading to better decision-making across the organization.
Their ability to detect anomalies faster allowed them to address issues proactively, minimizing potential damage and downtime.
They reduced their data-related compliance incidents, strengthening their regulatory standing and reducing potential fines or penalties.
Put together, these impacts gave FinTrust Bank’s data team new confidence in their analytics.
Best practices for distribution excellence
With the example of FinTrust Bank, it’s clear that mastering data distribution requires a comprehensive approach.
Start by aligning your distribution monitoring efforts with your business objectives: identify the critical metrics that need distribution validation, and define clear thresholds and response protocols. It’s critical to build consensus about acceptable ranges across stakeholders at this point, to ensure smooth implementation and adoption of the testing.
With your strategy set, build robust monitoring that uses automated checks and multiple statistical measures to validate your data’s behavior holistically. Don’t rely entirely on point-in-time checks; be sure to also monitor trends over time to spot gradual shifts in distribution patterns that might otherwise go unnoticed.
Integrating distribution checks into your CI/CD pipeline can help catch issues early in the development process.
Lastly, enable quick response mechanisms for when distribution issues are detected. Create clear escalation paths for distribution anomalies and maintain well-documented remediation procedures. Regular training on distribution management ensures that your team is equipped to handle issues as they arise. And by establishing feedback loops for continuous improvement, you can evolve your distribution management processes alongside your organization’s needs.
By following these best practices, you’ll be well-positioned to leverage data distribution analysis as a powerful tool for maintaining data quality and driving trusted analytics across your enterprise.
Common pitfalls to avoid
To avoid common pitfalls in distribution analysis, pay close attention to three key areas:
Avoid static thinking. Distributions aren’t constant. It’s important to account for seasonal and other cyclical changes in your data distributions, and make regular updates to your baselines accordingly.
Don’t oversimplify. Consider multimodal distributions, account for different business segments, and check for outliers that might be influencing your analysis.
Don’t work in isolation. Combine your distribution analysis with other dimensions of data quality for holistic data monitoring. Integrate your findings into your broader data management strategy, and share insights across departments to maximize the value of your efforts.
Looking ahead: The future of distribution analysis
As data volumes grow and analytical needs become more complex, organizations that master distribution-focused data quality monitoring will be better positioned for success. They’ll be able to make more reliable decisions with lower risk, while building stronger trust in their data internally and externally.
Excellence in data quality will continue to be a key differentiator for organizations, and distribution analysis is a critical part of that. By incorporating distribution-based data quality monitoring into your pipelines, you can position your organization for competitive success.
Join the conversation
How is your organization handling data distribution challenges? Join our community forum to share your experiences with other data practitioners whose teams are tackling similar problems. Together, we can build more robust approaches to data quality management.
Don’t miss the next installment of our series, where we’ll look at data integrity patterns and explore advanced techniques for ensuring data reliability across your enterprise.