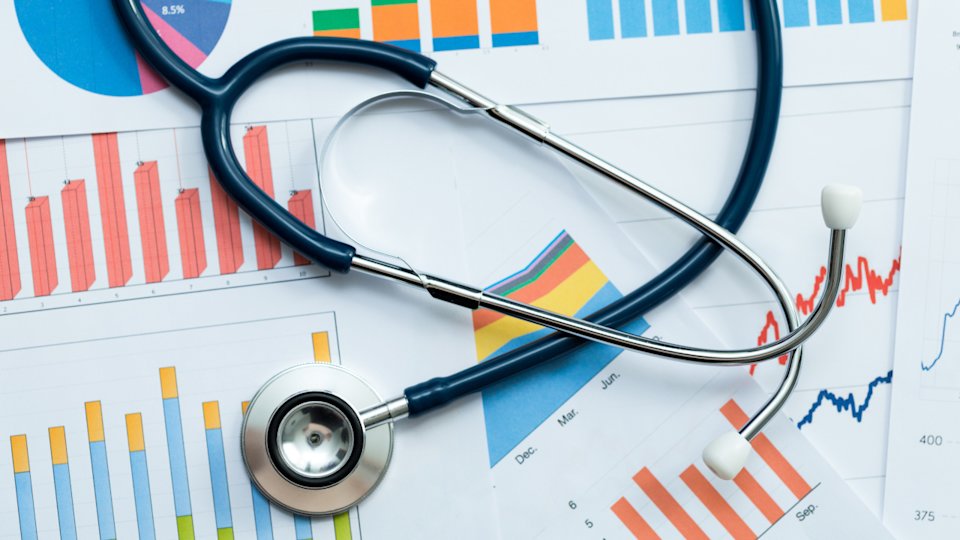
We all have workplace pet peeves. Someone takes up too much space in the communal fridge. Your coworker will not stop whistling. Your neighbor likes to rev up the lawnmower right when your team sync starts.
But no workplace annoyance is worse than something that makes it hard for you to do your job. And for data analysts, nothing makes the workday harder—or undermines your organization’s goals more—than poor data quality.
Data quality issues that go undetected or unaddressed can quickly cause a host of horror stories, as we covered on this blog recently.
We’re constantly talking to our customers and community about the data quality challenges they face. Here are the common symptoms of low data quality that data analysts find most troublesome.
1. Data from source systems keeps changing
Data from upstream sources, such as external vendors or internal systems that other teams manage, is subject to change—often without warning and beyond analysts’ control. These changes can wreak havoc on your data pipeline.
Say you receive regular reports of new customers from several of these upstream sources. Some of them format purchase dates differently from the rest—MM/DD/YY vs. DD/MM/YYYY, for example—and one of them even sends a file with two date columns, differently named and with different data.
Before an analyst can receive these reports, they’ll have to wait for someone to clean up all these inconsistencies, which can take quite a while.
If you’re an analyst who spends a lot of time waiting on data preparation and ingestion, that could be a major sign of low data quality in your pipeline.
Situations like these are strikingly common. In our research, nearly 40% of data analysts identified “changes from data in source systems (ex. changes in event types, ENUMs, valid codes, etc.)” as a top challenge for producing reliable, trusted data.
In some cases—our date format scenario being a good example—these changes are too small to completely break a pipeline. But that may actually be worse, as it allows the issue to fester undetected. By the time someone notices, data errors could have been compounding for days, weeks, or months.
2. The data is full of gaps
Incomplete datasets are one of the most common manifestations of poor data quality.
In fact, 35% of our surveyed data analysts selected “non-representative coverage,” a category that includes issues like missing object types, incorrect filters, and biased samples, as a top challenge for producing reliable and trusted data.
So what can that look like in practice? Maybe a few transactions go missing when a table is passed between two different systems. Maybe two transactions in the same table happen to be identical, and so one of them inadvertently gets lost to deduping—an especially plausible risk if some entries in the table are missing transaction ID numbers.
Whatever the particular issue, if a data analyst is working with incomplete data, they’re going to produce incomplete reports.
3. Teams disagree on definitions
What, exactly, constitutes a lead? Are they attributed to their first-touch channel, or to the link they clicked right before converting?
If questions like these aren’t answered in clear documentation, teams start creating their own answers, on their own terms, reflecting their own biases.
That leads to disconnects between teams, which in turn creates major headaches for the analysts working with the data those teams provide.
If you find this speaks to your own experience, you’re far from alone—more than one out of three respondents said that “creating a shared vocabulary around important concepts related to data” was one of their top challenges in collaborating with people outside of their immediate team.
4. People start going rogue
When the official tables and databases housing an organization’s data are a mess, individuals and teams across the company, naturally, don’t feel like using them. Instead, they start building and maintaining their own data repositories in places like Excel or Google Sheets.
These, of course, are governed by none of the standards or best practices that analysts rely on. Sometimes, analysts don’t even know these unofficial repositories exist.
Some data issues are hard to miss—a broken dashboard, for instance, is usually pretty obvious. But when data problems are more subtle, or occur farther upstream and out of sight, they can be more insidious, and go unaddressed until they’ve caused the headaches we’ve outlined here.
So what’s a data analyst to do? A good place to start is with a tool like GX Cloud, which you can configure to surface even the subtlest data quality issues before they ruin your day or upset your customers. Take a look at GX Cloud here.